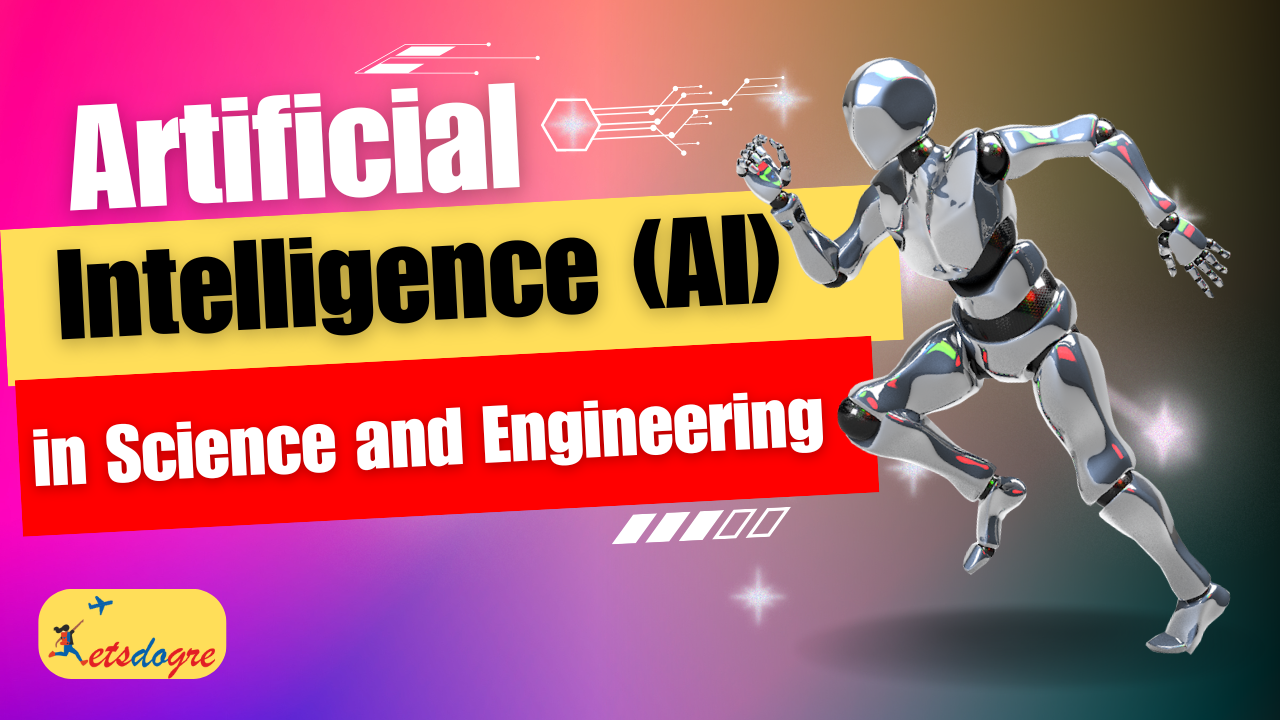
- April 6, 2024
- 12:50 pm
-
Views: 183
Artificial Intelligence (AI) in Science and Engineering
What is Artificial Intelligence?
AI entails the emulation of human cognitive processes by machines, predominantly computer systems. These processes encompass learning, logical deduction, problem-solving, perception, and decision-making. AI aims to create systems that can perform tasks that typically require human intelligence, such as understanding natural language, recognizing patterns, and adapting to new situations.
How does AI work in Advancing the Domains of Science and Engineering?
In recent years, the integration of AI has not just been a theoretical concept but a practical tool that has significantly reshaped the landscape of science and engineering. AI’s ability to analyze vast amounts of data, identify patterns, and make predictions has revolutionized various aspects of research and development across different disciplines, making it a crucial part of our daily scientific and engineering practices.
Enhanced Data Analysis and Pattern Recognition
One of the primary contributions of AI to science and engineering is its capability to handle complex datasets with unprecedented efficiency and accuracy, thereby accelerating the pace of research and development. Traditional methods often struggled to extract meaningful insights from large volumes of data. However, AI algorithms, particularly machine learning and deep learning models, excel at recognizing patterns and trends within datasets. This capability enhances researchers’ ability to analyze experimental results, identify correlations, and uncover hidden relationships, ultimately leading to novel discoveries and insights at a much faster rate.
Predictive Modeling and Simulation
AI-powered predictive modeling has emerged as a game-changer in numerous scientific and engineering domains. By leveraging historical data and computational algorithms, researchers can develop predictive models that forecast future outcomes with remarkable accuracy. In fields such as climate science, materials science, and drug discovery, AI models simulate complex systems, predict behaviors, and guide decision-making processes. These predictive capabilities enable scientists and engineers to anticipate phenomena, optimize designs, and mitigate risks more effectively.
Automation and Optimization:
Another significant benefit of AI in science and engineering is its capacity to automate repetitive tasks and optimize processes. From laboratory experiments to manufacturing operations, AI-driven automation streamlines workflows reduces manual labor and enhances efficiency. For instance, in bioinformatics, AI algorithms automate the analysis of genomic data, accelerating the identification of genetic markers and disease pathways. Similarly, in industrial engineering, AI-powered optimization algorithms improve production processes, minimize waste, and maximize resource utilization, leading to cost savings and increased productivity.

Facilitating Interdisciplinary Collaboration:
AI catalyzes interdisciplinary collaboration, bridging gaps between different scientific and engineering disciplines. By integrating diverse datasets and methodologies, AI enables researchers from various backgrounds to work together on complex problems. This interdisciplinary approach fosters innovation and synergy, leading to breakthroughs that would not be possible within individual silos. For example, AI techniques facilitate collaborations between computer scientists, biologists, and chemists in drug discovery, where diverse expertise is essential for developing new therapies and treatments.
Advantages of AI:
The benefits of artificial intelligence include increased efficiency, improved accuracy, enhanced productivity, personalized experiences, cost savings, faster innovation, better decision-making, and improved safety.
Examples of how Artificial Intelligence has Assisted in Advancements in Science and Technology
- Accelerating Drug Discovery with AI
Traditional drug discovery is a time-consuming and costly process that involves screening large libraries of compounds to identify potential candidates for further testing. However, AI has revolutionized this process by enabling researchers to analyze vast amounts of chemical and biological data, leading to the identification of promising drug candidates in a fraction of the time.
One notable example is the use of deep learning algorithms to predict the biological activity of molecules. By training neural networks on large databases of chemical structures and their corresponding biological activities, researchers can develop models that accurately predict how a new compound will interact with biological targets, such as proteins or enzymes. These AI models can prioritize compounds for experimental testing, significantly reducing the time and resources required for drug discovery.
Furthermore, AI-driven virtual screening techniques allow researchers to explore vast chemical space more efficiently, leading to the discovery of novel drug candidates with desirable properties. By leveraging machine learning algorithms to analyze structural features and properties of known drugs, AI can suggest modifications to existing molecules or propose entirely new chemical scaffolds with improved potency, selectivity, and safety profiles.
Overall, AI is revolutionizing drug discovery by accelerating the identification of promising drug candidates, optimizing lead optimization processes, and reducing the time and cost associated with bringing new therapies to market. This example illustrates how AI is transforming the pharmaceutical industry and unlocking new possibilities for treating diseases and improving human health.
- Optimizing Aircraft Design with AI
Aircraft design is a complex process that involves balancing numerous factors such as aerodynamics, structural integrity, fuel efficiency, and safety. Traditionally, designing an aircraft involves extensive computational simulations and physical testing, which could be time-consuming and costly. However, artificial intelligence is revolutionizing this process by enabling engineers to optimize aircraft designs more efficiently and effectively.
One example of AI’s impact on aircraft design is the use of generative design algorithms. Generative design involves specifying design goals and constraints and allowing AI algorithms to explore countless design possibilities, generating innovative solutions that human designers might not have considered. These algorithms leverage machine learning techniques to iteratively improve designs based on feedback and performance data iteratively, leading to more efficient and aerodynamic aircraft configurations.
Furthermore, AI is enhancing the performance of aircraft by enabling real-time optimization during flight. Advanced AI algorithms analyze sensor data, weather conditions, and aircraft performance metrics to continuously adjust flight parameters and optimize fuel consumption, reducing operating costs and environmental impact. Additionally, AI-powered predictive maintenance systems can anticipate component failures and schedule maintenance proactively, minimizing downtime and ensuring aircraft safety.
Overall, artificial intelligence is revolutionizing aircraft design and operations by enabling engineers to explore innovative design solutions, optimize performance, and enhance safety and efficiency. This example demonstrates how AI is transforming aerospace engineering and paving the way for the development of next-generation aircraft that are more sustainable, economical, and technologically advanced.
- AI-Assisted Writing in Scientific Research
Writing research articles and papers is a fundamental aspect of scientific communication. Still, it can be a time-consuming and challenging task, particularly for non-native English speakers or researchers with limited writing experience. However, artificial intelligence is increasingly being used to assist researchers in the writing process, helping them to produce clear, concise, and well-structured manuscripts.
One example of AI-assisted writing in scientific research is the use of natural language processing (NLP) tools to improve the clarity and coherence of scientific writing. These tools analyze the text to identify grammatical errors, improve sentence structure, and suggest alternative word choices to enhance readability. By leveraging AI-powered writing assistants, researchers can refine their manuscripts and ensure that their ideas are communicated effectively to the target audience.
Furthermore, AI algorithms can help researchers organize and synthesize large amounts of information from diverse sources, facilitating literature reviews and the formulation of research hypotheses. For example, AI-powered citation management tools can automatically generate bibliographies and format citations according to the required style guidelines, saving researchers valuable time and effort.
Additionally, AI-powered content generation tools can assist researchers in drafting sections of their manuscripts, such as abstracts, introductions, and conclusions, based on the analysis of existing research articles in the field. These tools can generate coherent and informative text tailored to the specific requirements of the manuscript, allowing researchers to focus on refining their ideas and conducting further experiments.
Overall, AI-assisted writing tools are empowering researchers to overcome language barriers, improve the quality of their manuscripts, and streamline the publication process. This example illustrates how AI is enhancing scientific communication by facilitating the creation of clear and compelling research articles and papers.
Conclusion
The integration of artificial intelligence has transformed the practice of science and engineering, empowering researchers to tackle complex challenges and unlock new opportunities for innovation. From data analysis and predictive modeling to automation and interdisciplinary collaboration, AI has become an indispensable tool in the researcher’s toolkit. As AI continues to evolve and mature, its impact on science and engineering will only grow, driving further advancements and revolutionizing how we understand and interact with the world around us.
Frequently Asked Questions
Artificial intelligence (AI) aims to create intelligent machines that can mimic human capabilities. These machines learn, reason, and even adjust their actions based on new information. AI systems tackle tasks traditionally requiring human smarts, like understanding what we see, recognizing speech, making decisions, and translating languages.AI technologies encompass machine learning, where algorithms are trained on data to improve performance over time, as well as neural networks, which attempt to mimic the structure and function of the human brain. AI has a wide range of applications across various industries, including healthcare, finance, transportation, and entertainment, and it continues to advance rapidly, raising both opportunities and challenges for society.
The future of Artificial Intelligence (AI) promises remarkable advancements across various sectors, driven by ongoing technological breakthroughs. AI will continue to automate tasks, enhance personalization, and foster collaboration between humans and machines. However, ethical concerns regarding privacy, bias, and accountability must be addressed to ensure responsible AI deployment. As AI becomes more integrated into daily life, its potential to revolutionize healthcare, sustainability efforts, and numerous other fields becomes increasingly evident, necessitating careful consideration of its societal impacts.
Artificial Intelligence (AI) and machine learning are closely related concepts within the field of computer science. AI is a broader discipline that aims to create intelligent systems capable of mimicking human intelligence to perform tasks such as problem-solving, decision-making, and language understanding. Machine learning is a powerful tool within AI. It allows computers to teach themselves by analyzing data. This lets them make predictions and decisions on new data without needing specific instructions for every situation. In other words, machine learning is a specific approach or technique used to achieve AI goals by allowing systems to learn and improve from experience. Therefore, machine learning is a crucial component of many AI applications, providing the capability for systems to adapt and evolve over time through the analysis of data
There are three major categories for classifying AI systems:
Narrow AI, General AI, and Artificial Superintelligence (ASI). Narrow AI is specialized in specific tasks within a limited domain, while General AI would possess human-like cognitive abilities and adaptability across various domains. ASI, a theoretical concept, represents AI systems with intelligence surpassing that of humans in all areas, raising significant ethical and existential questions.